Ethical Considerations in Machine Learning Development
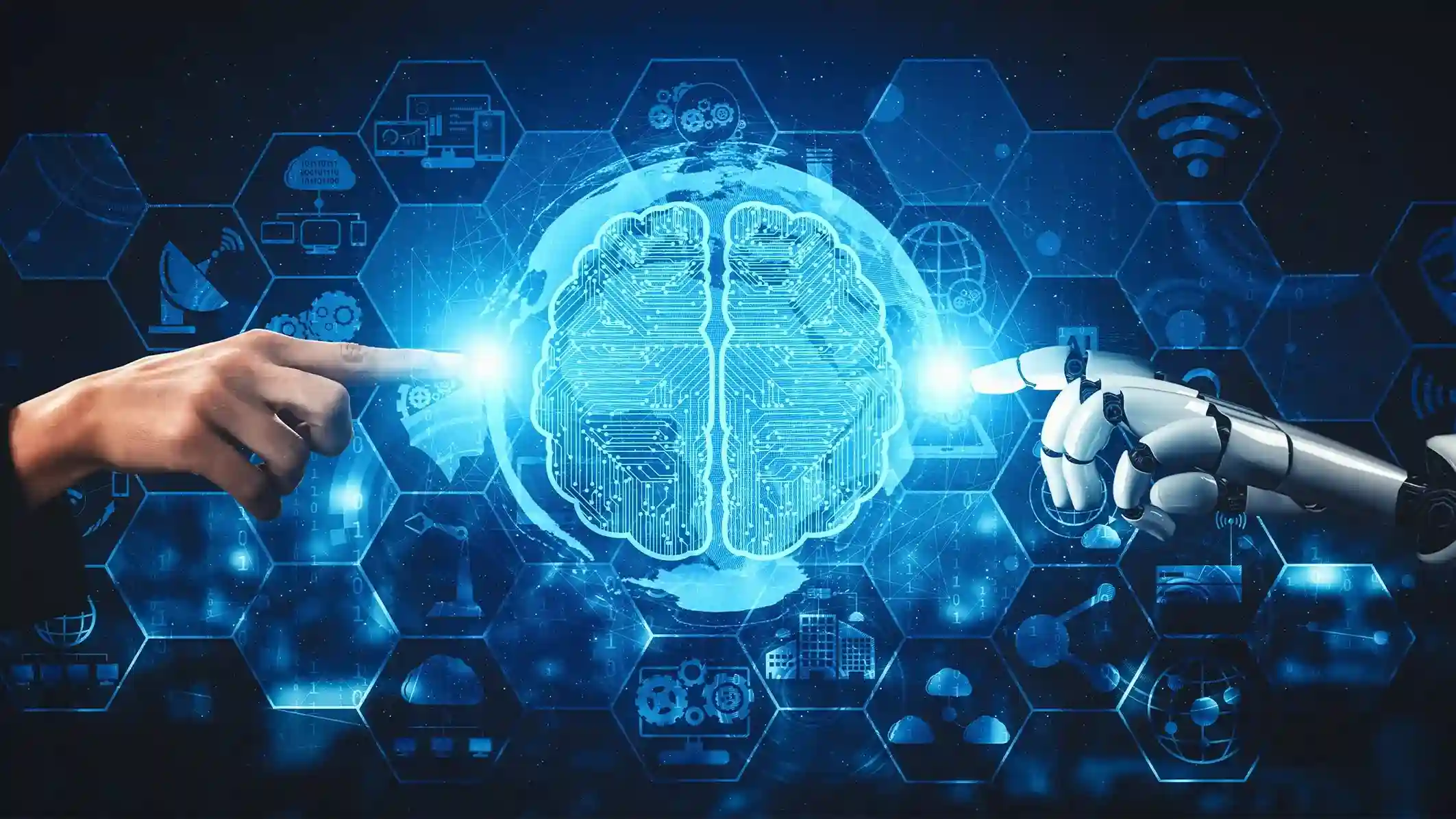
Machine learning (ML) development has brought about tremendous advancements across various industries, but it also raises important ethical considerations that developers and organizations must address. In this blog post, we’ll delve into the key ethical considerations in machine learning development and how they impact decision-making and societal well-being.
1. Bias and Fairness
Machine learning models can inadvertently perpetuate biases present in the training data, leading to unfair outcomes. Discuss:
- The importance of detecting and mitigating bias in ML algorithms.
- Strategies such as fairness-aware algorithms and diverse training data to promote fairness.
2. Transparency and Explainability
The “black box” nature of some ML models raises concerns about transparency and accountability. Cover:
- The need for transparent ML models that provide explanations for their decisions.
- Techniques like model interpretability and explainable AI (XAI) to enhance transparency.
3. Privacy and Data Security
ML systems often deal with sensitive user data, requiring robust privacy measures. Touch on:
- Data anonymization and encryption techniques to protect user privacy.
- Compliance with regulations like GDPR and CCPA regarding data handling and user consent.
4. Algorithmic Accountability
Who is responsible when ML algorithms make mistakes or cause harm? Discuss:
- The concept of algorithmic accountability and the role of developers, organizations, and regulators.
- Creating frameworks for accountability, including error analysis and feedback mechanisms.
5. Impact on Jobs and Society
ML advancements may lead to job displacement and societal impacts. Explore:
- The potential effects of automation on employment and the workforce.
- Strategies for reskilling and upskilling workers to adapt to AI-driven changes.
6. Ethical AI Governance
Establishing ethical guidelines and governance frameworks is crucial. Include:
- Best practices for ethical AI development, such as human oversight and continuous monitoring.
- Collaboration between technologists, ethicists, policymakers, and stakeholders in shaping ethical AI frameworks.
7. Environmental Impact
The computational demands of ML algorithms contribute to energy consumption and environmental impact. Address:
- Sustainable AI practices, including energy-efficient algorithms and data center optimizations.
- Balancing technological advancements with environmental responsibility.
8. Inclusivity and Diversity
Ensuring inclusivity and diversity in ML development is essential for avoiding biases and promoting fairness. Discuss:
- The importance of diverse teams and perspectives in ML development.
- Mitigating biases through inclusive data collection and algorithm design.
Conclusion
Wrap up the blog post by emphasizing the need for a holistic approach to machine learning development that prioritizes ethical considerations. Encourage readers to stay informed about emerging ethical guidelines and contribute to responsible AI innovation.
More Blog

Building Your Machine Learning Model

UI/UX Trends for Mobile Apps
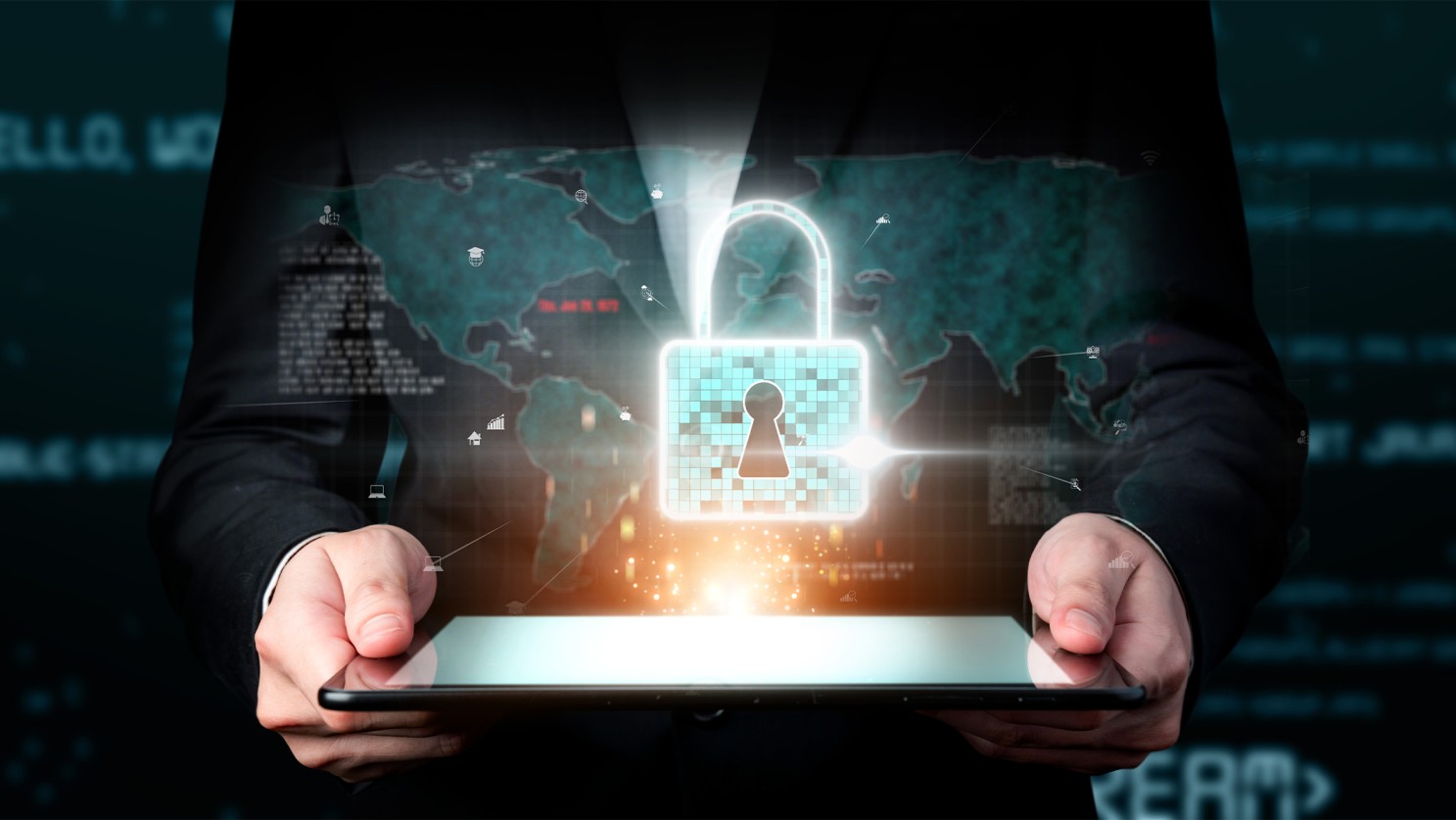